How I made an AI-generated show
At the beginning of this year, AI-generated content started gaining a lot of internet traction. From what I can recall, it all started with Nothing, Forever and quickly reached the peak of its popularity with AI Sponge (I just linked two whole Wikipedia pages. What?). If you’ve never heard of this kind of content, they are imaginary characters having short AI-generated conversations based on user inputs; you send a topic, and they briefly talk about it.
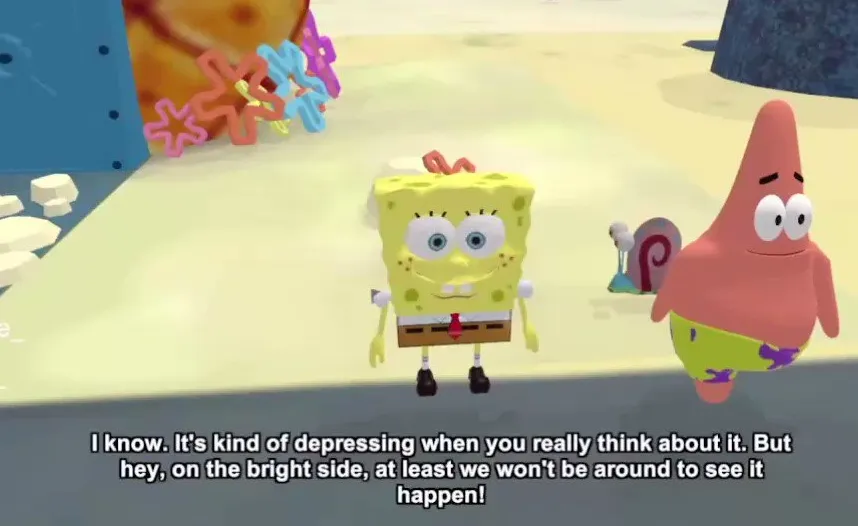
I find this type of content hilarious, and from the moment I watched the first livestream, I couldn’t resist the need to build something similar. In this post, I will explain how I did it, how it could be improved upon, and what roadblocks I had to face.
I’d also like to add that I’m not a game developer. The part I was interested in was mainly the back end, so this project couldn’t have been possible without the help of Micro, who made the actual front-end using Godot and was brave enough to work alongside me. He hasn’t published anything about it yet (and I don’t know if he’s ever going to), but I’ll try to keep this post up to date.
At first, we didn’t know what to actually build, but in the end, we decided to go with a parody of Rick and Morty, a brilliant show from Adult Swim (if you’ve never watched it, you should).
Update: CodeBullet recently published a video called “How I made AI Generated Rick and Morty Episodes”. He makes excellent content, and the video is entertaining; if you are interested in the topic, I recommend you watch it as he took a bit of a different approach from us.
Generating conversations based on prompts
To create an AI-generated show, we first needed a method to generate conversations from prompts and convert them into actual voices.
Before ChatGPT became a thing doing something like this would have been very complicated. Luckily OpenAI’s APIs simplified this process a lot, enabling me to accomplish what I was looking for through simple prompt engineering.
OpenAI offers a lot of models with different capabilities. The one I ended up picking was gpt-3.5-turbo
, at the time the most powerful and to this day the cheapest one, powering ChatGPT. There was also text-davici-003
(which was used by ai_sponge), but it wasn’t nearly as powerful and costed 10 times more.
Update: The text-davici-003
has been recently deprecated.
After the first setup, I only needed to find the right approach to generate conversations. Initially, I considered making multiple requests to ChatGPT, proceeding to attach the previous response to provide context. Conceptually, it would resemble the following:
# PROMPT 1
You need to act like Rick from "Rick and Morty".
Start a conversation about "<PROMPT>".
Your sentence has to be short, you are talking to "Morty".
# CHATGPT RESPONSE 1
[...]
# PROMPT 2
You need to act like Morty from "Rick and Morty" and respond to Rick.
Rick just told you "<CHATGPT RESPONSE>"
# CHATGPT RESPONSE 2
[...]
This approach had two issues, though:
- I had to start a new conversation every time. Otherwise, ChatGPT would get confused and hallucinate.
- I was making way too many requests to OpenAI, which was inefficient.
The second approach was to generate entire conversations with a single prompt like this:
# PROMPT
Simulate a conversation between Rick and Morty about "<PROMPT>".
# CHATGPT RESPONSE>
Rick: Sentence 1
Morty: Sentence 2
[...]
The only issue I encountered with this approach was that the formatting wasn’t always consistent. To circumvent this, I asked ChatGPT to send the response as a JSON. This method worked flawlessly 99% of the time, and as these edge cases were infrequent, failed requests would trigger a retry. If the retries failed twice consecutively – which had a very low likelihood of happening – the request was simply scrapped and logged.
Generating the voices
Now that I had a system to generate conversations, I needed to find a way to convert every sentence into an audio file. I couldn’t use standard text-to-speech (TTS) conversion as I wanted to use the actual voices used in the show.
I found many projects of this kind on GitHub, with Bark clearly being the most advanced one. Voiced generated by Bark are scary good; however, the developers had concerns about misuse, which led them to implement substantial restrictions against voice replication. While I did manage to find some workarounds, they were undocumented and challenging to implement. There were also other projects similar to Bark (TorToiSe being one of them), but in the end, I figured that it wasn’t worth the hustle as most of them weren’t good enough or had too many limitations (e.g., they only supported English).
Thanks to a YouTube video published by Fireship I also discovered ElevenLabs. ElevenLabs is impressive and uses a closed-sourced fork of TorToiSe under the hood. Unfortunately, it’s also expensive, costing a mindblowing $330 for ~40 hours of generated content. It’s not the best for a stream running 24/7 (it would have costed around $6138 each month!).
Luckily, I managed to find two alternatives: Uberduck and FakeYou. Both services have loads of pre-trained voice models, and while Uberduck was the most popular choice by a long shot, some of the voices I was looking for weren’t available.
Update: Uberduck seems to have deleted most of their pre-trained models. If you plan to build something like this, I recommend against using it.
So, I just went with FakeYou. Other than authentication (which had an endpoint that I only managed to find by reading the source code of one of their wrappers), their API is well-documented and easy to use.
After picking the right technology, everything was as easy as implementing a simple queue to convert the text to audio tracks, store them, and return them once the front end requests them. I built the queue with four statuses:
- QUEUED: Waiting in the queue. Another conversation is currently being generated.
- PENDING: The voices are being generated.
- DONE: The entire conversation is ready to be returned.
- READ: The conversation has been read and should be returned randomly if there aren’t any voices with the DONE status in the queue.
NOTE: If you plan to use FakeYou, remember that voices take a lot to generate (even if you have a paid plan). This problem can be circumvented by proxying the requests and starting multiple generations simultaneously.
Moderating content
Moderation was something important I didn’t consider at first while building the project. Allowing users to send whatever they want is dangerous, and staying online 24/7 to moderate wasn’t an option.
To fix this, I once again used the power of AI to give a rating to all prompts before being generated. If a prompt was deemed too explicit, it needed additional human review to pass.
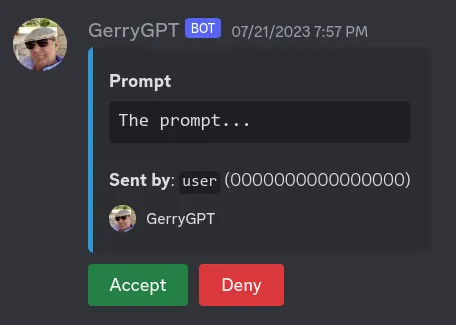
Going forward
The first proof of concept was incredibly fun to build and only took two days. If you want to see it in action you can find it on YouTube. Keep in mind that the content is pretty explicit as it still didn’t have any kind of moderation.
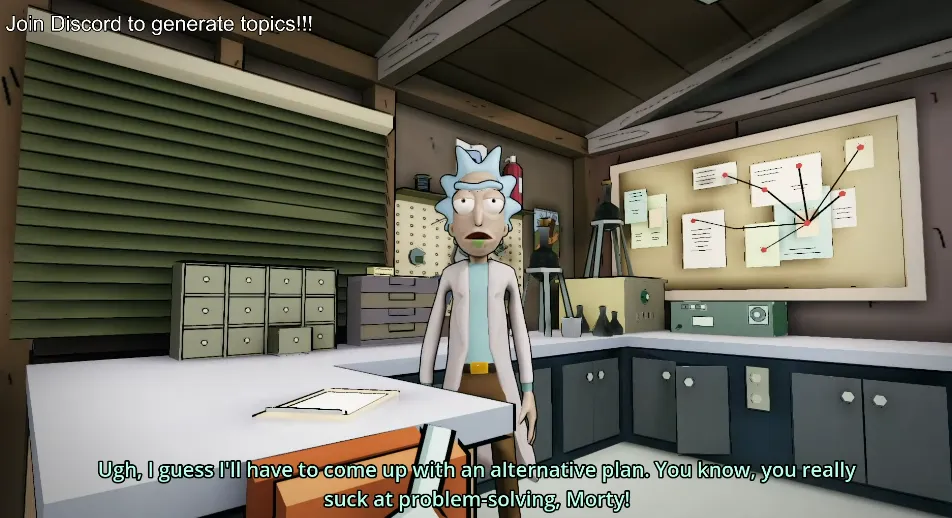
Once we were done with it, we created a new AI (this time in Italian, as we would have been the first ones in the country doing something of this kind) and called it GerryGPT. The project was much more polished, and we kept it running 24/7 for two months. It eventually became unsustainable, so unfortunately, we had to shut it down.
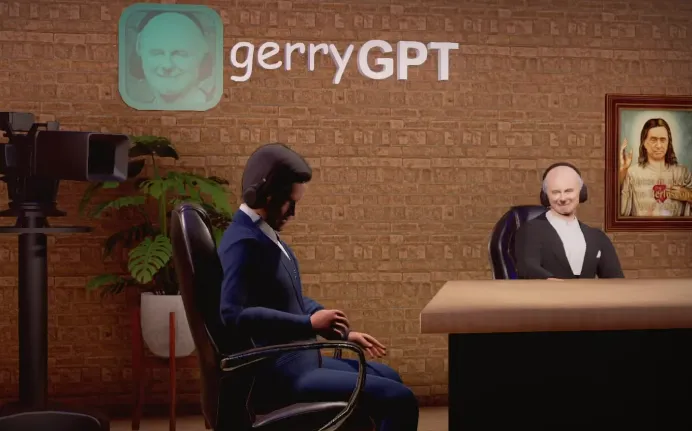
I loved building this whole project, allowing me to discover the potential of generative language models. I’ve got other things like it planned for the future. Stay tuned 🙂!